數(shù)據(jù)分析大佬用Python代碼教會你Mean Shift聚類
MeanShift算法可以稱之為均值漂移聚類,是基于聚類中心的聚類算法,但和k-means聚類不同的是,不需要提前設(shè)定類別的個數(shù)k。在MeanShift算法中聚類中心是通過一定范圍內(nèi)樣本密度來確定的,通過不斷更新聚類中心,直到最終的聚類中心達到終止條件。整個過程可以看下圖,我覺得還是比較形象的。
MeanShift向量
MeanShift向量是指對于樣本X1,在以樣本點X1為中心,半徑為h的高維球區(qū)域內(nèi)的所有樣本點X的加權(quán)平均值,如下所示,同時也是樣本點X1更新后的坐標(biāo)。
而終止條件則是指 | Mh(X) - X |<ε,滿足條件則樣本點X1停止更新,否則將以Mh(X)為新的樣本中心重復(fù)上述步驟。
核函數(shù)
核函數(shù)在機器學(xué)習(xí)(SVM,LR)中出現(xiàn)的頻率是非常高的,你可以把它看做是一種映射,是計算映射到高維空間之后的內(nèi)積的一種簡便方法。在這個算法中將使用高斯核,其函數(shù)形式如下。
h表示帶寬,當(dāng)帶寬h一定時,兩個樣本點距離越近,其核函數(shù)值越大;當(dāng)兩個樣本點距離一定時,h越大,核函數(shù)值越小。核函數(shù)代碼如下,gaosi_value為以樣本點X1為中心,半徑為h的高維球范圍內(nèi)所有樣本點與X1的高斯核函數(shù)值,是一個(m,1)的矩陣。
def gaussian_kernel(self,distant): m=shape(distant)[1]#樣本數(shù) gaosi=mat(zeros((m,1))) for i in range(m): gaosi[i][0]=(distant.tolist()[0][i]*distant.tolist()[0][i]*(-0.5)/(self.bandwidth*self.bandwidth)) gaosi[i][0]=exp(gaosi[i][0]) q=1/(sqrt(2*pi)*self.bandwidth) gaosi_value=q*gaosi return gaosi_value
MeanShift向量與核函數(shù)
在01中有提到MeanShift向量是指對于樣本X1,在以樣本點X1為中心,半徑為h的高維球區(qū)域內(nèi)的所有樣本點X的加權(quán)平均值。但事實上是不同點對于樣本X1的貢獻程度是不一樣的,因此將權(quán)值(1/k)更改為每個樣本與樣本點X1的核函數(shù)值。改進后的MeanShift向量如下所示。
其中
就是指高斯核函數(shù),Sh表示在半徑h內(nèi)的所有樣本點集合。
MeanShift算法原理
在MeanShift算法中實際上利用了概率密度,求得概率密度的局部最優(yōu)解。
對于一個概率密度函數(shù)f(x),已知一個概率密度函數(shù)f(X),其核密度估計為
其中K(X)是單位核,概率密度函數(shù)f(X)的梯度估計為
其中G(X)=-K'(X)。第一個中括號是以G(X)為核函數(shù)對概率密度的估計,第二個中括號是MeanShift 向量。因此MeanShift向量是與概率密度函數(shù)的梯度成正比的,總是指向概率密度增加的方向。
而對于MeanShift向量,可以將其變形為下列形式,其中mh(x)為樣本點X更新后的位置。
MeanShift算法流程
在未被標(biāo)記的數(shù)據(jù)點中隨機選擇一個點作為起始中心點X;
找出以X為中心半徑為radius的區(qū)域中出現(xiàn)的所有數(shù)據(jù)點,認為這些點同屬于一個聚類C。同時在該聚類中記錄數(shù)據(jù)點出現(xiàn)的次數(shù)加1。
以X為中心點,計算從X開始到集合M中每個元素的向量,將這些向量相加,得到向量Mh(X)。
mh(x) =Mh(X) + X。即X沿著Mh(X)的方向移動,移動距離是||Mh(X)||。
重復(fù)步驟2、3、4,直到Mh(X)的很小(就是迭代到收斂),記住此時的X。注意,這個迭代過程中遇到的點都應(yīng)該歸類到簇C。
如果收斂時當(dāng)前簇C的center與其它已經(jīng)存在的簇C2中心的距離小于閾值,那么把C2和C合并,數(shù)據(jù)點出現(xiàn)次數(shù)也對應(yīng)合并。否則,把C作為新的聚類。
重復(fù)1、2、3、4、5直到所有的點都被標(biāo)記為已訪問。
分類:根據(jù)每個類,對每個點的訪問頻率,取訪問頻率最大的那個類,作為當(dāng)前點集的所屬類。
TIPS:每一個樣本點都需要計算其漂移均值,并根據(jù)計算出的漂移均值進行移動,直至滿足終止條件,最終得到的均值漂移點為該點的聚類中心點。
MeanShift算法代碼
from numpy import *from matplotlib import pyplot as plt
class mean_shift(): def __init__(self): #帶寬 self.bandwidth=2 #漂移點收斂條件 self.mindistance=0.001 #簇心距離,小于該值則兩簇心合并 self.cudistance=2.5
def gaussian_kernel(self,distant): m=shape(distant)[1]#樣本數(shù) gaosi=mat(zeros((m,1))) for i in range(m): gaosi[i][0]=(distant.tolist()[0][i]*distant.tolist()[0][i]*(-0.5)/(self.bandwidth*self.bandwidth)) gaosi[i][0]=exp(gaosi[i][0]) q=1/(sqrt(2*pi)*self.bandwidth) gaosi_value=q*gaosi return gaosi_value
def load_data(self): X =array([ [-4, -3.5], [-3.5, -5], [-2.7, -4.5], [-2, -4.5], [-2.9, -2.9], [-0.4, -4.5], [-1.4, -2.5], [-1.6, -2], [-1.5, -1.3], [-0.5, -2.1], [-0.6, -1], [0, -1.6], [-2.8, -1], [-2.4, -0.6], [-3.5, 0], [-0.2, 4], [0.9, 1.8], [1, 2.2], [1.1, 2.8], [1.1, 3.4], [1, 4.5], [1.8, 0.3], [2.2, 1.3], [2.9, 0], [2.7, 1.2], [3, 3], [3.4, 2.8], [3, 5], [5.4, 1.2], [6.3, 2],[0,0],[0.2,0.2],[0.1, 0.1],[-4, -3.5]]) x,y=[],[] for i in range(shape(X)[0]): x.a(chǎn)ppend(X[i][0]) y.a(chǎn)ppend(X[i][1]) plt.scatter(x,y,c='r') # plt.plot(x, y) plt.show() classlable=mat(zeros((shape(X)[0],1))) return X,classlable
def distance(self,a,b): v=a-b return sqrt(v*mat(v).T).tolist()[0][0] def shift_point(self,point,data,clusterfrequency): sum=0 n=shape(data)[0] ou=mat(zeros((n,1))) t=mat(zeros((n,1))) newdata=[] for i in range(n): # print(self.distance(point,data[i])) d=self.distance(point,data[i]) if d<self.bandwidth: ou[i][0] =d t[i][0]=1 newdata.a(chǎn)ppend(data[i]) clusterfrequency[i]=clusterfrequency[i]+1 gaosi=self.gaussian_kernel(ou[t==1]) meanshift=gaosi.T*mat(newdata) return meanshift/gaosi.sum(),clusterfrequency
def group2(self,dataset,clusters,m): data=[] fre=[] for i in clusters: i['data']=[] fre.a(chǎn)ppend(i['frequnecy']) for j in range(m): n=where(array(fre)[:,j]==max(array(fre)[:,j]))[0][0] data.a(chǎn)ppend(n) clusters[n]['data'].a(chǎn)ppend(dataset[j]) print("一共有%d個簇心" % len(set(data))) # print(clusters) # print(data) return clusters
def plot(self,dataset,clust): colors = 10 * ['r', 'g', 'b', 'k', 'y','orange','purple'] plt.figure(figsize=(5, 5)) plt.xlim((-8, 8)) plt.ylim((-8, 8)) plt.scatter(dataset[:, 0],dataset[:, 1], s=20) theta = linspace(0, 2 * pi, 800) for i in range(len(clust)): cluster = clust[i] data = array(cluster['data']) if len(data): plt.scatter(data[:, 0], data[:, 1], color=colors[i], s=20) centroid =cluster['centroid'].tolist()[0] plt.scatter(centroid[0], centroid[1], color=colors[i], marker='x', s=30) x, y = cos(theta) * self.bandwidth + centroid[0], sin(theta) * self.bandwidth + centroid[1] plt.plot(x, y, linewidth=1, color=colors[i]) plt.show()
def mean_shift_train(self): dataset, classlable = self.load_data() m = shape(dataset)[0] clusters = [] for i in range(m): max_distance = inf cluster_centroid = dataset[i] # print(cluster_centroid) cluster_frequency =zeros((m,1)) while max_distance>self.mindistance: w,cluster_frequency = self.shift_point(cluster_centroid,dataset,cluster_frequency) dis = self.distance(cluster_centroid, w) if dis < max_distance: max_distance = dis # print(max_distance) cluster_centroid = w has_same_cluster = False for cluster in clusters: if self.distance(cluster['centroid'],cluster_centroid)<self.cudistance: cluster['frequnecy']=cluster['frequnecy']+cluster_frequency has_same_cluster=True break if not has_same_cluster: clusters.a(chǎn)ppend({'frequnecy':cluster_frequency,'centroid':cluster_centroid}) clusters=self.group2(dataset,clusters,m) print(clusters) self.plot(dataset,clusters)
if __name__=="__main__": shift=mean_shift() shift.mean_shift_train()
得到的結(jié)果圖如下。
之后還會詳細解說K-means聚類以及DBSCAN聚類,敬請關(guān)注。
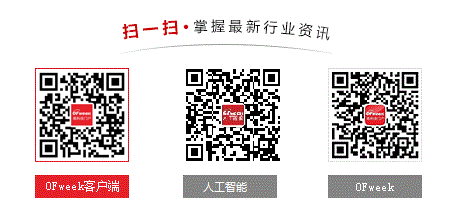
請輸入評論內(nèi)容...
請輸入評論/評論長度6~500個字
最新活動更多
-
即日-10.29立即報名>> 2024德州儀器嵌入式技術(shù)創(chuàng)新發(fā)展研討會
-
10月31日立即下載>> 【限時免費下載】TE暖通空調(diào)系統(tǒng)高效可靠的組件解決方案
-
即日-11.13立即報名>>> 【在線會議】多物理場仿真助跑新能源汽車
-
11月14日立即報名>> 2024工程師系列—工業(yè)電子技術(shù)在線會議
-
12月19日立即報名>> 【線下會議】OFweek 2024(第九屆)物聯(lián)網(wǎng)產(chǎn)業(yè)大會
-
即日-12.26火熱報名中>> OFweek2024中國智造CIO在線峰會
推薦專題
- 1 Intel宣布40年來最重大轉(zhuǎn)型:年底前裁員15000人、拋掉2/3房產(chǎn)
- 2 因美封殺TikTok,字節(jié)股價骨折!估值僅Meta1/5
- 3 宏山激光重磅發(fā)布行業(yè)解決方案,助力智能制造產(chǎn)業(yè)新飛躍
- 4 國產(chǎn)AI芯片公司破產(chǎn)!白菜價拍賣
- 5 具身智能火了,但規(guī)模落地還需時間
- 6 國產(chǎn)英偉達們,抓緊沖刺A股
- 7 三次錯失風(fēng)口!OpenAI前員工殺回AI編程賽道,老東家捧金相助
- 8 英特爾賦能智慧醫(yī)療,共創(chuàng)數(shù)字化未來
- 9 英偉達的麻煩在后頭?
- 10 將“網(wǎng)紅”變成“商品”,AI“爆改”實力拉滿
- 高級軟件工程師 廣東省/深圳市
- 自動化高級工程師 廣東省/深圳市
- 光器件研發(fā)工程師 福建省/福州市
- 銷售總監(jiān)(光器件) 北京市/海淀區(qū)
- 激光器高級銷售經(jīng)理 上海市/虹口區(qū)
- 光器件物理工程師 北京市/海淀區(qū)
- 激光研發(fā)工程師 北京市/昌平區(qū)
- 技術(shù)專家 廣東省/江門市
- 封裝工程師 北京市/海淀區(qū)
- 結(jié)構(gòu)工程師 廣東省/深圳市